電氣工程及其自動化 外文翻譯 外文文獻(xiàn) 英文文獻(xiàn) 建立一個自動車輛車牌識別系統(tǒng)
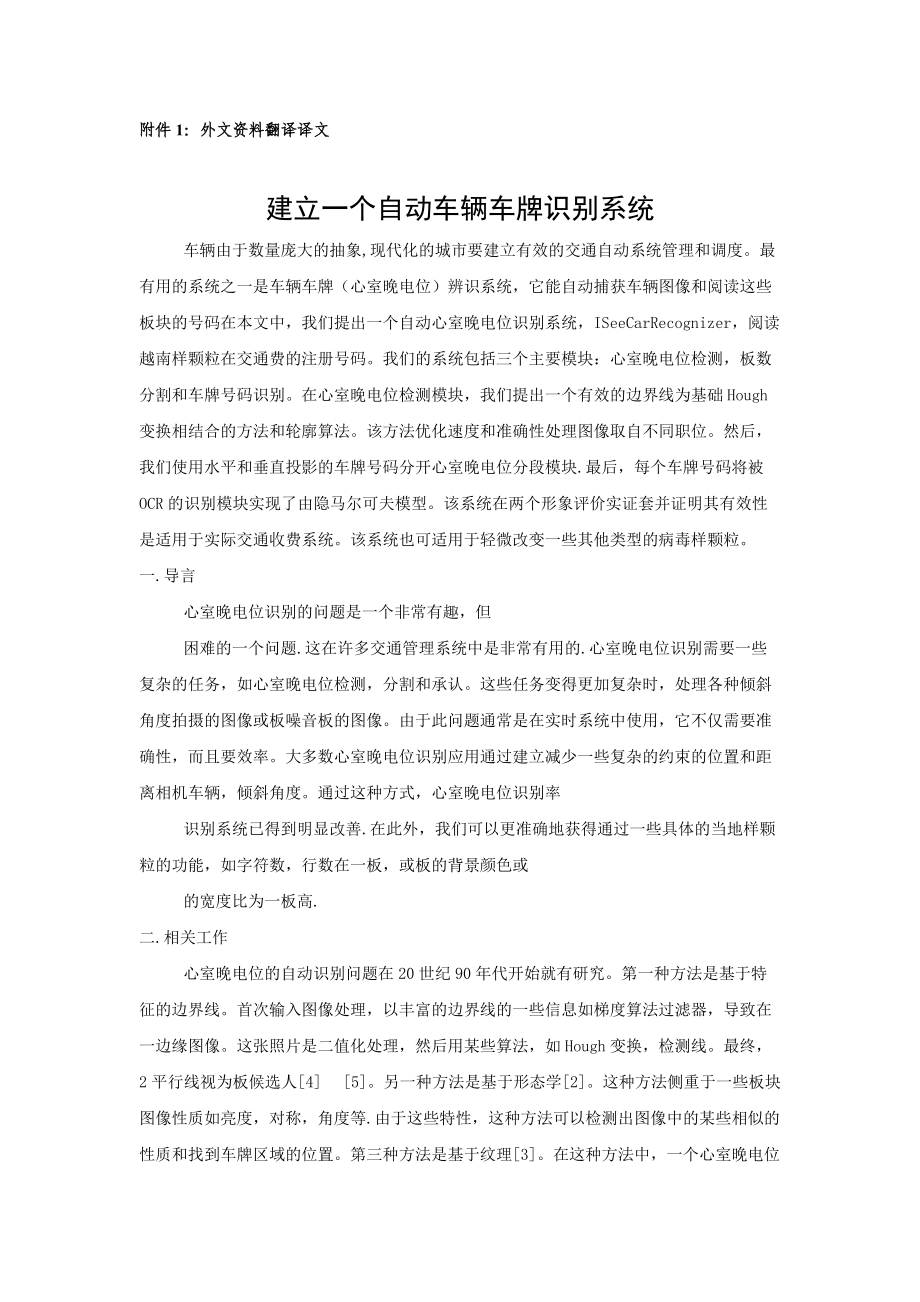


《電氣工程及其自動化 外文翻譯 外文文獻(xiàn) 英文文獻(xiàn) 建立一個自動車輛車牌識別系統(tǒng)》由會員分享,可在線閱讀,更多相關(guān)《電氣工程及其自動化 外文翻譯 外文文獻(xiàn) 英文文獻(xiàn) 建立一個自動車輛車牌識別系統(tǒng)(9頁珍藏版)》請在裝配圖網(wǎng)上搜索。
1、附件1:外文資料翻譯譯文 建立一個自動車輛車牌識別系統(tǒng) 車輛由于數(shù)量龐大的抽象,現(xiàn)代化的城市要建立有效的交通自動系統(tǒng)管理和調(diào)度。最有用的系統(tǒng)之一是車輛車牌(心室晚電位)辨識系統(tǒng),它能自動捕獲車輛圖像和閱讀這些板塊的號碼在本文中,我們提出一個自動心室晚電位識別系統(tǒng),ISeeCarRecognizer,閱讀越南樣顆粒在交通費的注冊號碼。我們的系統(tǒng)包括三個主要模塊:心室晚電位檢測,板數(shù)分割和車牌號碼識別。在心室晚電位檢測模塊,我們提出一個有效的邊界線為基礎(chǔ)Hough變換相結(jié)合的方法和輪廓算法。該方法優(yōu)化速度和準(zhǔn)確性處理圖像取自不同職位。然后,我們使用水平和垂直投影的車牌號碼分開心室晚電位分段
2、模塊.最后,每個車牌號碼將被OCR的識別模塊實現(xiàn)了由隱馬爾可夫模型。該系統(tǒng)在兩個形象評價實證套并證明其有效性是適用于實際交通收費系統(tǒng)。該系統(tǒng)也可適用于輕微改變一些其他類型的病毒樣顆粒。 一.導(dǎo)言 心室晚電位識別的問題是一個非常有趣,但 困難的一個問題.這在許多交通管理系統(tǒng)中是非常有用的.心室晚電位識別需要一些復(fù)雜的任務(wù),如心室晚電位檢測,分割和承認(rèn)。這些任務(wù)變得更加復(fù)雜時,處理各種傾斜角度拍攝的圖像或板噪音板的圖像。由于此問題通常是在實時系統(tǒng)中使用,它不僅需要準(zhǔn)確性,而且要效率。大多數(shù)心室晚電位識別應(yīng)用通過建立減少一些復(fù)雜的約束的位置和距離相機(jī)車輛,傾斜角度。通過這種方式,心室晚電位識
3、別率 識別系統(tǒng)已得到明顯改善.在此外,我們可以更準(zhǔn)確地獲得通過一些具體的當(dāng)?shù)貥宇w粒的功能,如字符數(shù),行數(shù)在一板,或板的背景顏色或 的寬度比為一板高. 二.相關(guān)工作 心室晚電位的自動識別問題在20世紀(jì)90年代開始就有研究。第一種方法是基于特征的邊界線。首次輸入圖像處理,以豐富的邊界線的一些信息如梯度算法過濾器,導(dǎo)致在一邊緣圖像。這張照片是二值化處理,然后用某些算法,如Hough變換,檢測線。最終,2平行線視為板候選人[4] [5]。另一種方法是基于形態(tài)學(xué)[2]。這種方法側(cè)重于一些板塊圖像性質(zhì)如亮度,對稱,角度等.由于這些特性,這種方法可以檢測出圖像中的某些相似的性質(zhì)和找到車牌區(qū)域的位置。
4、第三種方法是基于紋理[3]。在這種方法中,一個心室晚電位被認(rèn)為是一個對象和不同的紋理幀。大小不同的紋理窗框用于檢測板的候選人。每個人獲得通過一個分類,以確認(rèn)它是否是一個盤子或沒有。這常用的方法是尋找圖像中的文字任務(wù)。此外,已經(jīng)出現(xiàn)了一些其他有關(guān)這個問題的方法上注重檢測心室晚電位 在視頻數(shù)據(jù)。 三.擬議的系統(tǒng) 我們的系統(tǒng),ISeeCarRecognizer,由四個模塊:前處理,心室晚電位檢測,字符分割,和光學(xué)字符識別(OCR),在其中最后三個模塊處理三個主要問題一個心室晚電位識別域。是VLP檢測模塊接收到的圖像有被處理的預(yù)處理模塊 -第一個輸入該系統(tǒng)的模塊。這個模塊的結(jié)果圖像發(fā)送到分段模塊
5、。分割段模塊板的圖像,成為獨立的characterimages。這些字符的圖像,然后認(rèn)可光學(xué)字符識別模塊和最終結(jié)果是ASCII字符和板塊中的數(shù)字。 1.預(yù)處理 從相機(jī)拍攝的圖像進(jìn)行處理的預(yù)處理模塊。本模塊的目的是豐富的邊緣特征。由于我們的檢測方法在邊界上的基地功能,它可以改善成功率的心室晚電位檢測模塊。該算法在此模塊順序使用的老齡化,規(guī)范化和直方圖均衡。在得到一個灰階圖片中,我們使用過濾器來提取索貝爾邊緣圖像,然后以一個二進(jìn)制閾值的一個圖像。我們用于局部自適應(yīng)閾值算法二值化的一步。特別是,我們發(fā)展一種基于動態(tài)規(guī)劃,優(yōu)化其速度,使其適用于實時應(yīng)用[1]。圖像的結(jié)果被用作心室晚電位檢測模塊的輸
6、入。 2.心室晚電位檢測算法 在邊界為基礎(chǔ)的方法,最重要的步驟是檢測邊界線。最有效的算法之一是Hough變換申請?zhí)崛〉亩M(jìn)制映像線從對象的圖像。然后我們找兩平行線,其包含的區(qū)域被認(rèn)為platecandidates。然而,這種方法的缺點是,霍夫變換的執(zhí)行時間需要太多多的運(yùn)算時,被應(yīng)用到一個二進(jìn)制圖像與大量的像素。特別是,較大的圖像慢的算法。該算法的速度可能會通過細(xì)化圖像改進(jìn),然后再應(yīng)用霍夫變換。然而,細(xì)化算法也慢。這種限制使這種方法不適合實時交通管理系統(tǒng)。該算法在本系統(tǒng)中我們采用的是組合 Hough變換的算法和輪廓產(chǎn)生更高的精度和更快的速度,它可以適用于實時系統(tǒng)。 1)結(jié)合Hough變換
7、和輪廓算法心室晚電位檢測 我們的做法是:從提取的邊緣圖片中,我們使用封閉的輪廓檢測算法邊界的對象。這些輪廓線改造到霍夫協(xié)調(diào),找到兩個平行線互動(2 -平行線之一成立回另兩平行線并建立一個平行四邊形表對象)是作為板候選人考慮。由于有相當(dāng)少(黑)在等高線的像素,轉(zhuǎn)化這些需要協(xié)調(diào)霍夫點少得多計算。因此,該算法的速度提高沒有明顯的精度損失。然而,有些板塊可能會覆蓋眼鏡或裝飾燈。這些對象還可能有 形狀兩個相互作用二平行線,因此是錯誤地檢測為板候選人。要拒絕這樣不正確的候選人,我們評估一個模塊的實施無論候選人是板或沒有。 2)板考生核查 從兩個候選人的水平線,我們可以如何準(zhǔn)確地計算出它從水平傾斜坐
8、標(biāo)。然后,我們應(yīng)用旋轉(zhuǎn)轉(zhuǎn)換調(diào)整它為平角。經(jīng)過處理,這些標(biāo)準(zhǔn)二進(jìn)制板候選區(qū)域被傳遞給一個號碼啟發(fā)式檢測和評估算法。我們的評價板候選人在兩個算法基地主要步驟,分別采取。這兩個步驟是:
(1)評價之間的高度和寬度的比例候選人,
(2)使用水平橫切來計算數(shù)切入候選人的對象。
我們只選擇了檢查和候選人有寬度與高度之比滿足預(yù)先定義約束:minWHRatio<寬/高 9、橫切。在這個階段,我們使用兩個水平削減和再算上該由這些橫切削減對象的數(shù)量。一候選人將被視為一個盤子,如果數(shù)量的減少選擇對象為每個板塊在一定范圍內(nèi)適當(dāng)
通過實驗類型。這個數(shù)字必須在數(shù)量大致范圍在一類病毒顆粒的字符,我們有兩個合適的約束兩個越南板類型:預(yù)處理OCR的分割拍攝的圖像心室晚電位檢測從相機(jī)許可證帕泰字符:4≤1≤8排板候選人7≤2≤n時排板候選人16與N是禁對象的數(shù)量。候選人是滿足了上述兩個約束選定為最終結(jié)果。在我們的制度,我們實施的1 /3兩hoziontal削減和2 / 3的板候選人的高度。平均的數(shù)目切對象將被計算。這種評估有助于確定正確的板候選人。
(3).分割
要正確認(rèn)識字 10、,我們要一車牌圖像二值圖像的設(shè)置只包含一車牌字符。這些形象將被傳遞到對于OCR的識別模塊。這個任務(wù)常見的算法是運(yùn)用預(yù)測。然而,在一些情況下,無法正常工作。我們現(xiàn)在將描述我們在分割方法添加一些增強(qiáng)此方法。
我們用一個水平投影檢測和部分行排在二板。因為二進(jìn)制圖像進(jìn)行了調(diào)整板他們的傾斜角度為零,分割結(jié)果的行幾乎是完美的。與最低值的位置水平投影是啟動或在最后一排板。不同形式的行分割,字符分割更為困難,因為許多原因,如卡字符,螺絲,和泥覆蓋板。這些噪音事情的原因使用的字符分割算法垂直投影,有一些錯誤。在一些最嚴(yán)重的圖像質(zhì)量差板的情況下,一個字符可以分割成兩部分。我們應(yīng)用的若干制約因素比到一個字符的寬度 11、高度。我們尋求的最低值在垂直 投影,只有這給削減最低位置 件滿足所有預(yù)定義的限制被認(rèn)為是
字符分割點。通過此增強(qiáng),我們在這項任務(wù)中取得了較好的效果。經(jīng)過這一步,我們有一個人物候選人名單。并不是所有的考生實際上是人物形象。
到那時,我們可以重新評估候選人是否板是一盤或不檢查的字符數(shù)候選人。在越南,一盤只包含7或8字符。最后一盤的候選人,連同與他們的字符列表傳遞到OCR模塊負(fù)責(zé)確認(rèn)。
(4).用于光學(xué)字符識別隱馬爾可夫模型
在這個系統(tǒng)中,我們使用的字符的HMM模型承認(rèn)。我們的特點,在此模型中使用的在窗口中的比例前景像素。我們使用的9 9大小的窗口,這個掃描在圖像窗口中,從左至右,從上到下 12、
這些窗口可以由兩個互相重疊三分之二的大小。通過這種方式,我們有一個特征向量其中包括196值。
在識別模塊,我們需要一個字符分類成一個形象的36個班(26個英文字母:甲,乙,丙...
和10個數(shù)字字符:0,1,2 ...)。要培養(yǎng)我們的模型,我們使用的訓(xùn)練,是從圖像中提取套
病毒樣顆粒。對每類樣本數(shù)約為60.These提取樣品圖像實時心室晚電位一點點的噪音,所以在良好的訓(xùn)練,該模型可以正是認(rèn)識到板的同類型的噪音。在最后一步,我們使用越南的一些具體規(guī)則病毒樣顆粒以提高準(zhǔn)確性。我們了解到,第三次在車牌字符必須是字母,四是有時信,但通常是一個數(shù)字,其他位置當(dāng)然是數(shù)字。
四.實證評價
我們 13、的系統(tǒng)進(jìn)行了評價與越南兩個套車輛的車牌。圖像由索尼DC350數(shù)碼相機(jī),具有800x600像素大小,在不同地點和時間。我們使用Microsoft Visual C++ 6.0,運(yùn)行惠普工作站X2000奔騰IV,1.4千兆赫,512 MB的的RAM,Windows XP操作系統(tǒng)。
五.結(jié)論和未來工作
該系統(tǒng)運(yùn)行良好的越南各類 心室晚電位的圖像,甚至抓傷,縮放板的圖像。在 此外,它可以處理多個板塊中的案件 相同的圖像,或不同類型的車輛,如摩托車 板,汽車板或車板。然而,它仍然有幾個 錯誤在處理劣質(zhì)板材。
我們正在數(shù)的算法在預(yù)處理模塊。其目的是探測地區(qū)的第一盤地區(qū)可能,從而減少 計算成本的心室晚 14、電位檢測算法。在 此外,我們打算結(jié)合的紋理為基礎(chǔ)的數(shù) 方法,和機(jī)器學(xué)習(xí)的方法來評價platecadidates。我們相信,這些將提高信息的準(zhǔn)確性和該算法的速度進(jìn)一步。
索引詞:車載車牌識別,實時系統(tǒng),Hough變換,輪廓算法。
附件2:外文原文(復(fù)印件)
Building an Automatic Vehicle License-Plate
Recognition System
Abstract—Due to a huge number of vehicles, modern cities need to establish effecti 15、vely automatic systems for traffic management and scheduling.One of the most useful systems is the Vehicle License-Plate (VLP) Recognition System which captures images of vehicles and read these plates’ registration numbers automatically.In this paper, we present an automatic VLP Recognition System, 16、 ISeeCarRecognizer, to read Vietnamese VLPs’ registration numbers at traffic tolls.Our system consists of three main modules: VLP detection, plate number segmentation, and plate number recognition.In VLP detection module, we propose an efficient boundary line-based method combining the Hough transfo 17、rm and Contour algorithm.This method optimizes speed and accuracy in processing images taken from various positions. Then, we use horizontal and vertical projection to separate plate numbers in VLP segmentation module.Finally, each plate number will be recognized by OCR module implemented by Hidden 18、Markov Model.The system was evaluated in two empirical image sets and has proved its effectiveness (see section IV) which is
applicable in real traffic toll systems. The system can also be applied to some other types of VLPs with minor changes.
I. INTRODUCTION
The problem of VLP recognition is 19、a very interesting butdifficult one.It is very useful for many trafficmanagement systems.VLP recognition requires some complex tasks, such as VLP detection, segmentation and recognition.These tasks become more sophisticated when dealing with plate images taken in various inclined angles orplate imag 20、es with noise.Because this problem is usually used in real-time systems, it requires not only accuracy but also fast processing.Most VLP recognition applications reduce the complexity by establishing some constrains on the position and distance from the camera to vehicles, and the inclined angles.By 21、 that way, the recognition rate of VLP recognition systems has been improved significantly.In addition, we can gain more accuracy by using some specific features of local VLPs, such as the number of characters, the
number of rows in a plate, or colors of plate background, or the ratio of width to h 22、eight of a plate .
II. RELATED WORK
The problem of automatic VLP recognition has been
studied since 1990s.The first approach was based on characteristics of boundary lines.The input image was first processed to enrich boundary lines’ information by some algorithms such as the gradient filter, a 23、nd resulted in an edging image.This image was binarized and then processed by certain algorithms, such as Hough transform, to detect lines.Eventually, couples of 2-parallel lines were considered as a plate-candidate [4][5].Another approach was morphology-based [2].This approach focuses on some prope 24、rties of plate images such as their brightness,symmetry, angles, etc.Due to these properties, this method
can detect the similar properties in a certain image and locate the position of license plate regions.The third approach was texture-based [3].In this approach, a VLP was considered as an objec 25、t with different textures and frames.The texture window frames of different sizes were
used to detect plate-candidates.Each candidate was passed to a classifier to confirm whether it is a plate or not.This approach was commonly used in finding text in images tasks.In addition, there have been a num 26、ber of other methods relating to this problem focusing on detecting VLP in video data.
III. THE PROPOSED SYSTEM
Our system, ISeeCarRecognizer, consists of four
modules: Pre-processing, VLP detection, character
segmentation, and optical character recognition (OCR), in which the last three modul 27、es deal with three main problems of a VLP recognition domain.The VLP detection module receives images which have been processed by the preprocessing module – the first input module of this system.The resulted images of this module are sent to the segmentation module.The segmentation
module segments 28、 plate-images into separate characterimages.These character-images are then recognized by the OCR module and the final results are ASCII characters andnumbers in plates.
A. Preprocessing
Images taken from camera were processed by the preprocessing module.The purpose of this module was to enrich 29、the edge features.Because our detection method bases on the boundary features, it will improve the successful rate of the VLP detection module.The algorithms sequentially used in this module are graying, normalizing and histogram equalization.After having obtained a greyscale image, we use Sobel fil 30、ters to extract the edging image, and then thresholds the image to a binary one.We used the local adaptive thresholding algorithm for the binarization step.Especially, we develop an algorithm based
on dynamic programming to optimize its speed and make it suitable to real-time applications [1].The r 31、esulted images are used as inputs for the VLP detection module.
B. VLP Detection Algorithm
In boundary-based approach, the most important step is to detect boundary lines.One of most efficient algorithms is Hough transform applying to the binary image to extract lines from object-images.Then we 32、look for two parallel lines, whose the contained region is considered platecandidates.However, the drawback of this approach is that the execution time of the Hough transform requires too much computation when being applied to a binary image with great number of pixels.Especially, the larger image t 33、he
slower the algorithm is. The speed of the algorithm may beimproved by thinning image before applying the Houghtransform.Nevertheless, the thinning algorithm is also slow. This limitation makes the approach unsuitable for real time
traffic management systems.The algorithm we used in this system 34、is the combination of the Hough Transform and Contour algorithm which produces higher accuracy and faster speed so that it can be applied to real time systems.
1) Combine Hough Transform and Contour Algorithm for Detecting VLP
Our approach is as follows: from the extracted edging image, we use t 35、he contour algorithm to detect closed boundaries of objects.considered as a plate-candidate.Since there are quite few
(black) pixels in the contour lines, the transformation of
these points to Hough coordinate required much lesscomputation. Hence, the speed of the algorithm is improved significant 36、ly without the loss of accuracy .
However, some plates may be covered by glasses ordecorated with headlights.These objects may also have the shape of two interacted 2-parallel lines, and therefore, arealso falsely detected as plate-candidates. To reject suchincorrect candidates, we implement a modu 37、le for evaluating whether a candidate is a plate or not.
2) Plate-Candidates Verification
From the two horizontal lines of a candidate, we can calculate exactly how inclined it was from horizontal coordinate. Then we apply a rotate transformation to adjust it to straight angle. After processed, 38、these straight binary
plate-candidate regions were passed to a number of
heuristics and algorithms for evaluating.Our evaluating plate-candidates algorithm bases on two
main steps, which are taken respectively. The two steps are:(a) evaluate the ratios between the heights and the widths of the ca 39、ndidates, (b) use horizontal crosscuts to count the number of cut-objects in the candidates.In this stage, we check and only select out candidates that have the ratios of width to height satisfying pre-defined constraint: minWHRatio < W/H < maxWHRatioSince there are two main types of Vietnamese plat 40、es: 1-row and 2-row , we have two adequate constraints for two types.3.5 < W/H < 4.5 with 1-row plate-candidates0.8 < W/H < 1.4 with 2-row plate-candidatesThose candidates which satisfied one of the two aboveconstraints are selected and passed to the nextevaluation.Evaluate by using horizontal cross 41、cutsIn this stage, we use two horizontal cuts and then count the number of objects that are cut by these crosscuts.
A candidate will be considered as a plate if the number of cut objects is in the given range chosen suitably for each plate type by experiments .This number must be in the approximate 42、 range of the number of characters in a VLP, we have two appropriate constraints for two types of Vietnamese plates:Preprocessing OCR Segmentation.
Images taken VLP Detection from camera License-patecharacters:4 ≤ N ≤ 8 with 1-row plate-candidates7 ≤ N ≤ 16 with 2-row plate-candidates
With N is 43、the number of cut-objects.The candidates that satisfied one of the two above constraints are selected as the final result.
In our system, we implemented two hoziontal cuts at 1/3and 2/3 of plate-candidate’s height. The average of number of cut objects will be calculated. This evaluation helps to id 44、entify the correct plate-candidates.
C. Segmentation
To correctly recognize characters, we have to segment a binary plate image to set of images which only contain one license character. These character images will be passed to the OCR module for recognizing. The common algorithm for this task i 45、s applying projections. However, in some cases, it does not work correctly. We will now describe our approach in segmentation by adding some enhancements to this method.
We use a horizontal projection to detect and segment rows in 2 row plates. Because binary plate images were adjustedtheir incli 46、ned angles to zero, the result of row segmentation is nearly perfect. The positions with minimum values of horizontal projection are the start or the end of a row in plate.
Different form row segmentation, character segmentation is more difficult due to many reasons such as stuck characters, scre 47、ws, and mud covered in plates. These noise things cause the character segmentation algorithm using vertical projection to have some mistakes. In some worst cases of bad quality plate images, a character can be segmented into two pieces.We apply several constraints of
ratio of the height to the widt 48、h of a character.
We search for the minimum values in the vertical projection and only the minimum positions which give cut pieces satisfied all predefined constraints are considered as the points for character segmentation. By this enhancement,we have achieved better results in this task. After 49、this step,we have a list of character candidates. Not all of the candidates are actually images of characters.
By that time, we can re-evaluate whether a plate candidate is a plate or not by checking the number of characters of candidates. In Vietnam, a plate contains only 7 or 8 characters . The f 50、inal plate candidates, together with their list of characters are passed to the OCR module for recognizing.
D. Hidden Markov Model for OCR
In this system, we use the HMM model for character
recognition. The features which we used in this model are the ratio of foreground pixels in a window.
We 51、 use a window with the size of 99, and scan this window in the image from left to right and top to bottom These windows can overlap each other by two thirds of their size. By this way, we have a feature vector which includes 196 values.
In the recognition module, we need to classify a character i 52、mage into one of 36 classes (26 alphabet letters: A, B, C…and 10 numeric characters: 0, 1, 2…).To train our model, we use training sets which were extracted from images of
VLPs.The number of samples for every class is about60.These samples were extracted from real VLP images with a little noise, so 53、 after well trained, the model can recognize exactly plates with the similar types of noise.In the last step, we use some specific rules of Vietnamese VLPs to improve accuracy.We learned that the third character in plate must be a letter, the fourth is sometimes a letter but usually a number, and th 54、e other positions are surely numbers.
IV. EMPIRICAL EVALUATION
Our system was evaluated with two sets of Vietnamese vehicles’ plates. Images were taken by a Sony DC350 digital camera, with size of 800x600 pixels, in different places and times. We use Microsoft Visual C++ 6.0, run on HP Workstati 55、on X2000 Pentium IV, 1.4 GHz, 512 MB RAM, Windows XP OS.
V. CONCLUSIONS AND FUTURE WORK
The system performs well on various types of Vietnamese VLP images, even on scratched, scaled plate images. In addition, it can deal with the cases of multiple plates in the same image, or different types of 56、vehicles such as motorbike
plates, car plates or truck plates. However, it still has a few errors when dealing with bad quality plates.
We are working on a number of algorithms in the preprocessing module. The purpose is to detect regions that are likely plate regions first and thus to reduce th 57、e computation cost of the VLP detection algorithm. In addition, we intend to combine a number of texture-based approachs, and machine learning methods to evaluate platecadidates. We believe these will improve the accuracy and the speed of the algorithm furthermore.
Index Terms—Vehicle License-Plate Recognition, Real-time
System, Hough Transform, Contour Algorithm.
- 溫馨提示:
1: 本站所有資源如無特殊說明,都需要本地電腦安裝OFFICE2007和PDF閱讀器。圖紙軟件為CAD,CAXA,PROE,UG,SolidWorks等.壓縮文件請下載最新的WinRAR軟件解壓。
2: 本站的文檔不包含任何第三方提供的附件圖紙等,如果需要附件,請聯(lián)系上傳者。文件的所有權(quán)益歸上傳用戶所有。
3.本站RAR壓縮包中若帶圖紙,網(wǎng)頁內(nèi)容里面會有圖紙預(yù)覽,若沒有圖紙預(yù)覽就沒有圖紙。
4. 未經(jīng)權(quán)益所有人同意不得將文件中的內(nèi)容挪作商業(yè)或盈利用途。
5. 裝配圖網(wǎng)僅提供信息存儲空間,僅對用戶上傳內(nèi)容的表現(xiàn)方式做保護(hù)處理,對用戶上傳分享的文檔內(nèi)容本身不做任何修改或編輯,并不能對任何下載內(nèi)容負(fù)責(zé)。
6. 下載文件中如有侵權(quán)或不適當(dāng)內(nèi)容,請與我們聯(lián)系,我們立即糾正。
7. 本站不保證下載資源的準(zhǔn)確性、安全性和完整性, 同時也不承擔(dān)用戶因使用這些下載資源對自己和他人造成任何形式的傷害或損失。
最新文檔
- 市教育局冬季運(yùn)動會安全工作預(yù)案
- 2024年秋季《思想道德與法治》大作業(yè)及答案3套試卷
- 2024年教師年度考核表個人工作總結(jié)(可編輯)
- 2024年xx村兩委涉案資金退還保證書
- 2024年憲法宣傳周活動總結(jié)+在機(jī)關(guān)“弘揚(yáng)憲法精神推動發(fā)改工作高質(zhì)量發(fā)展”專題宣講報告會上的講話
- 2024年XX村合作社年報總結(jié)
- 2024-2025年秋季第一學(xué)期初中歷史上冊教研組工作總結(jié)
- 2024年小學(xué)高級教師年終工作總結(jié)匯報
- 2024-2025年秋季第一學(xué)期初中物理上冊教研組工作總結(jié)
- 2024年xx鎮(zhèn)交通年度總結(jié)
- 2024-2025年秋季第一學(xué)期小學(xué)語文教師工作總結(jié)
- 2024年XX村陳規(guī)陋習(xí)整治報告
- 2025年學(xué)校元旦迎新盛典活動策劃方案
- 2024年學(xué)校周邊安全隱患自查報告
- 2024年XX鎮(zhèn)農(nóng)村規(guī)劃管控述職報告